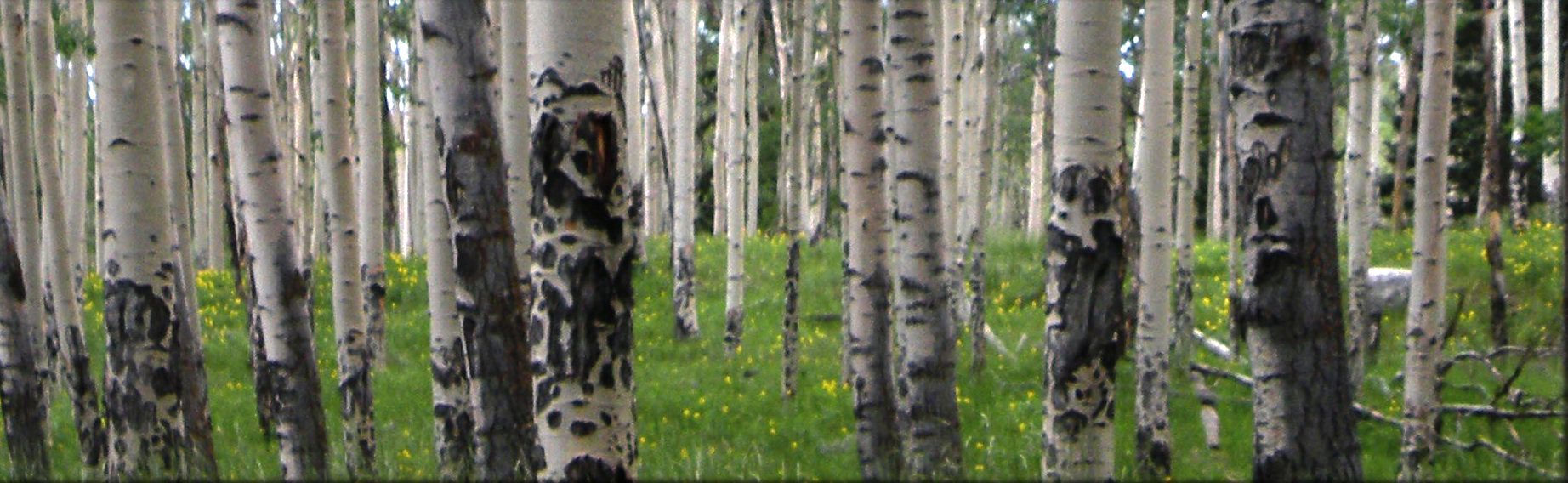
Aspen Bibliography
Estimating Tree Species Composition From Airborne Laser Scanning Data Using Point-Based Deep Learning Models
Document Type
Article
Author ORCID Identifier
Brent A. Murray https://orcid.org/0000-0003-3053-9448
Nicholas C. Coops https://orcid.org/0000-0002-0151-9037
Lukas Winiwarter https://orcid.org/0000-0001-8229-1160
Joanne C. White https://orcid.org/0000-0003-4674-0373
Adam Dick https://orcid.org/0000-0002-6802-2842
Journal/Book Title/Conference
ISPRS Journal of Photogrammetry and Remote Sensing
Volume
207
Publisher
Elsevier BV
First Page
282
Last Page
297
Publication Date
1-2024
Abstract
Airborne laser scanning (ALS) data is increasingly being used for accurate estimations of forest inventory attributes, such as tree height and volume. However, determining tree species information, an important attribute required by inventories for a range of forest management applications, is challenging to extract from ALS data. This is due to complex species assemblages and vertical structures in certain forest environments, and a lack of spectral information for most ALS sensors. Our objective was to estimate tree species compositions in a Canadian boreal forest environment using ALS data and point-based deep learning techniques. To accomplish this, we utilised existing polygonal forest resource information to develop a large comprehensive dataset of tree species compositions across a 630,000 ha forest management area. We then used an adapted PointAugment generative adversarial network (GAN) coupled with the dynamic graph convolutional neural network to estimate tree species proportions. This innovative deep learning approach resulted in an overall R2adj of 0.61 for all tree species proportions. A weighted F1 score of 63% was observed when classifying the leading species of a plot, 66% for leading genus, and 85% when distinguishing between coniferous and deciduous dominated plots. Furthermore, the PointAugment GAN successfully generated augmented point clouds of forest plots which can alleviate issues associated with limited training samples for use in deep learning. This approach demonstrates the capability of point-based deep learning techniques to accurately estimate tree species compositions from ALS point clouds within an expansive forested region, characterized by a complex management history and a diversity of tree species. The source code along with the pretrained models are available at https://github.com/Brent-Murray/point-dl/tree/main/Pytorch/models/PointAugment.
Recommended Citation
Murray, B. A., N. C. Coops, L. Winiwarter, J. C. White, A. Dick, I. Barbeito, and A. Ragab. 2024. Estimating tree species composition from airborne laser scanning data using point-based deep learning models. ISPRS Journal of Photogrammetry and Remote Sensing 207:282-297.