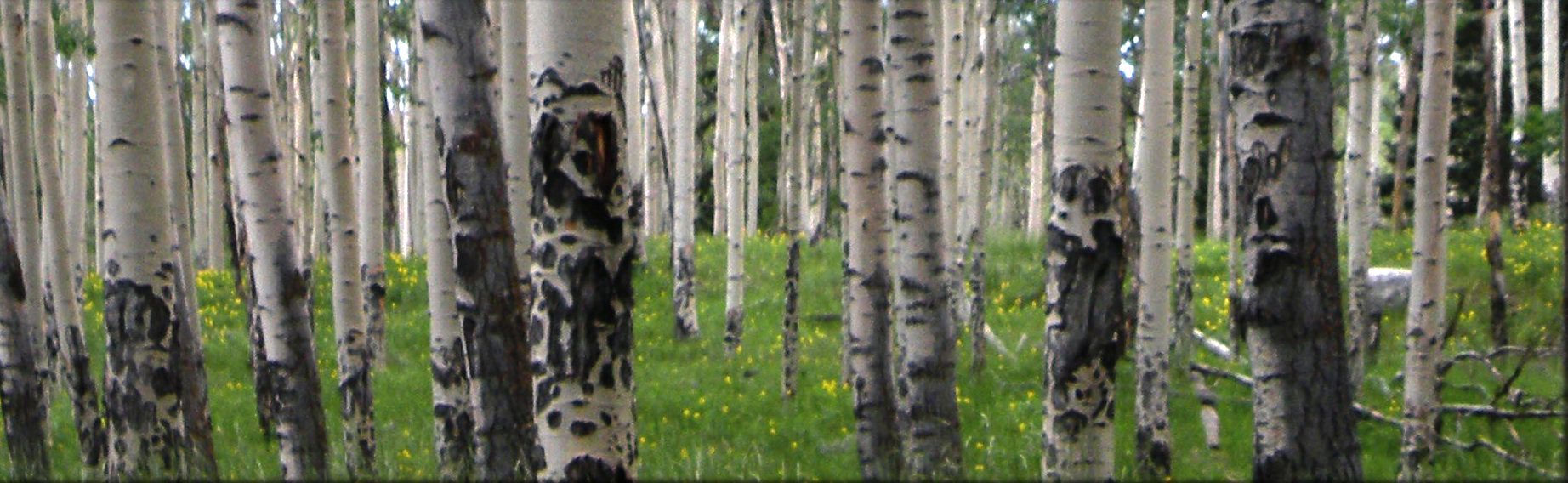
Aspen Bibliography
Enhancing Forest Insect Outbreak Detection by Integrating Tree-Ring and Climate Variables
Document Type
Article
Journal/Book Title/Conference
Journal of Forestry Research
Volume
35
Publisher
Northeast Forestry University * Chinese Association of Zoological Gardens, Dongbei Linye Daxue
First Page
1
Last Page
15
Publication Date
7-2-2024
Abstract
Annual tree rings are widely recognized as valuable tools for quantifying and reconstructing historical forest disturbances. However, the influence of climate can complicate the detection of disturbance signals, leading to limited accuracy in existing methods. In this study, we propose a random under-sampling boosting (RUB) classifier that integrates both tree-ring and climate variables to enhance the detection of forest insect outbreaks. The study focused on 32 sites in Alberta, Canada, which documented insect outbreaks from 1939 to 2010. Through thorough feature engineering, model development, and tenfold cross-validation, multiple machine learning (ML) models were constructed. These models used ring width indices (RWIs) and climate variables within and 11-year window as input features, with outbreak and non-outbreak occurrences as the corresponding output variables. Our results reveal that the RUB model consistently demonstrated superior overall performance and stability, with an accuracy of 88.1%, which surpassed that of the other ML models. In addition, the relative importance of the feature variables followed the order RWIs > mean maximum temperature (Tmax) from May to July > mean total precipitation (Pmean) in July > mean minimum temperature (Tmin) in October. More importantly, the dfoliatR (an R package for detecting insect defoliation) and curve intervention detection methods were inferior to the RUB model. Our findings underscore that integrating tree-ring width and climate variables as predictors in machine learning offers a promising avenue for enhancing the accuracy of detecting forest insect outbreaks.
Recommended Citation
Jiang, Y., Wang, Z., Zhang, Z. et al. Enhancing forest insect outbreak detection by integrating tree-ring and climate variables. J. For. Res. 35, 106 (2024). https://doi.org/10.1007/s11676-024-01759-x