Document Type
Article
Author ORCID Identifier
Yagnashree Velanki https://orcid.org/0009-0009-9460-3286
Pouya Hosseinzadeh https://orcid.org/0000-0001-8045-2709
Soukaina Filali Boubrahimi https://orcid.org/0000-0001-5693-6383
Shah Muhammad Hamdi https://orcid.org/0000-0002-9303-7835
Journal/Book Title/Conference
Universe
Volume
10
Issue
9
Publisher
MDPI AG
Publication Date
9-19-2024
Journal Article Version
Version of Record
First Page
1
Last Page
17
Creative Commons License
This work is licensed under a Creative Commons Attribution 4.0 License.
Abstract
Solar flares are significant occurrences in solar physics, impacting space weather and terrestrial technologies. Accurate classification of solar flares is essential for predicting space weather and minimizing potential disruptions to communication, navigation, and power systems. This study addresses the challenge of selecting the most relevant features from multivariate time-series data, specifically focusing on solar flares. We employ methods such as Mutual Information (MI), Minimum Redundancy Maximum Relevance (mRMR), and Euclidean Distance to identify key features for classification. Recognizing the performance variability of different feature selection techniques, we introduce an ensemble approach to compute feature weights. By combining outputs from multiple methods, our ensemble method provides a more comprehensive understanding of the importance of features. Our results show that the ensemble approach significantly improves classification performance, achieving values 0.15 higher in True Skill Statistic (TSS) values compared to individual feature selection methods. Additionally, our method offers valuable insights into the underlying physical processes of solar flares, leading to more effective space weather forecasting and enhanced mitigation strategies for communication, navigation, and power system disruptions.
Recommended Citation
Velanki, Y.; Hosseinzadeh, P.; Boubrahimi, S.F.; Hamdi, S.M. Time-Series Feature Selection for Solar Flare Forecasting. Universe 2024, 10, 373. https://doi.org/10.3390/universe10090373
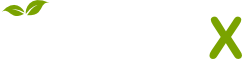
- Citations
- Citation Indexes: 3
- Usage
- Downloads: 47
- Abstract Views: 11
- Captures
- Readers: 2
- Mentions
- Blog Mentions: 1