Document Type
Article
Author ORCID Identifier
Akhila Akkala https://orcid.org/0009-0005-8016-7533
Soukaina Filali Boubrahimi https://orcid.org/0000-0001-5693-6383
Shah Muhammad Hamdi https://orcid.org/0000-0002-9303-7835
Pouya Hosseinzadeh https://orcid.org/0000-0001-8045-2709
Ayman Nassar https://orcid.org/0000-0003-0878-5861
Journal/Book Title/Conference
Hydrology
Volume
12
Issue
3
Publisher
MDPI AG
Publication Date
3-17-2025
Journal Article Version
Version of Record
First Page
1
Last Page
27
Creative Commons License
This work is licensed under a Creative Commons Attribution 4.0 License.
Abstract
Streamflow prediction is vital for effective water resource management, enabling a better understanding of hydrological variability and its response to environmental factors. This study presents a spatio-temporal graph neural network (STGNN) model for streamflow prediction in the Upper Colorado River Basin (UCRB), integrating graph convolutional networks (GCNs) to model spatial connectivity and long short-term memory (LSTM) networks to capture temporal dynamics. Using 30 years of monthly streamflow data from 20 monitoring stations, the STGNN predicted streamflow over a 36-month horizon and was evaluated against traditional models, including random forest regression (RFR), LSTM, gated recurrent units (GRU), and seasonal auto-regressive integrated moving average (SARIMA). The STGNN outperformed these models across multiple metrics, achieving an R2 of 0.78, an RMSE of 0.81 mm/month, and a KGE of 0.79 at critical locations like Lees Ferry. A sequential analysis of input–output configurations identified the (36, 36) setup as optimal for balancing historical context and forecasting accuracy. Additionally, the STGNN showed strong generalizability when applied to other locations within the UCRB. These results underscore the importance of integrating spatial dependencies and temporal dynamics in hydrological forecasting, offering a scalable and adaptable framework to improve predictive accuracy and support adaptive water resource management in river basins.
Recommended Citation
Akkala, A.; Boubrahimi, S.F.; Hamdi, S.M.; Hosseinzadeh, P.; Nassar, A. Spatio-Temporal Graph Neural Networks for Streamflow Prediction in the Upper Colorado Basin. Hydrology 2025, 12, 60. https://doi.org/10.3390/ hydrology12030060
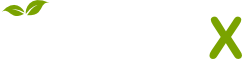
- Usage
- Downloads: 7
- Abstract Views: 3
- Captures
- Readers: 1
- Mentions
- Blog Mentions: 1
- News Mentions: 1