Document Type
Article
Journal/Book Title/Conference
Applied Sciences
Publisher
MDPI AG
Publication Date
12-8-2020
First Page
1
Last Page
19
Creative Commons License
This work is licensed under a Creative Commons Attribution-Noncommercial 4.0 License
Abstract
Turbulence and attenuation are signal degrading factors that can severely hinder free-space and underwater OAM optical pattern demultiplexing. A variety of state-of-the-art convolutional neural network architectures are explored to identify which, if any, provide optimal performance under these non-ideal environmental conditions. Hyperparameter searches are performed on the architectures to ensure that near-ideal settings are used for training. Architectures are compared in various scenarios and the best performing, with their settings, are provided. We show that from the current state-of-the-art architectures, DenseNet outperforms all others when memory is not a constraint. When memory footprint is a factor, ShuffleNet is shown to performed the best.
Recommended Citation
Neary, Patrick L.; Watnik, Abbie T.; Judd, Kyle Peter; Lindle, James R.; and Flann, Nicholas S., "CNN Classification Architecture Study for Turbulent Free-Space and Attenuated Underwater Optical OAM Communications" (2020). Space Dynamics Laboratory Publications. Paper 265.
https://digitalcommons.usu.edu/sdl_pubs/265
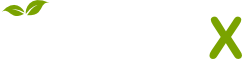
- Citations
- Citation Indexes: 13
- Usage
- Downloads: 5
- Abstract Views: 1
- Captures
- Readers: 8
- Mentions
- Blog Mentions: 1