Document Type
Article
Journal/Book Title/Conference
PLoS One
Volume
14
Issue
10
Publisher
Public Library of Science
Publication Date
10-31-2019
First Page
1
Last Page
14
Creative Commons License
This work is licensed under a Creative Commons Attribution 4.0 License.
Abstract
The accuracy of four machine learning methods in predicting narrative macrostructure scores was compared to scores obtained by human raters utilizing a criterion-referenced progress monitoring rubric. The machine learning methods that were explored covered methods that utilized hand-engineered features, as well as those that learn directly from the raw text. The predictive models were trained on a corpus of 414 narratives from a normative sample of school-aged children (5;0-9;11) who were given a standardized measure of narrative proficiency. Performance was measured using Quadratic Weighted Kappa, a metric of inter-rater reliability. The results indicated that one model, BERT, not only achieved significantly higher scoring accuracy than the other methods, but was consistent with scores obtained by human raters using a valid and reliable rubric. The findings from this study suggest that a machine learning method, specifically, BERT, shows promise as a way to automate the scoring of narrative macrostructure for potential use in clinical practice.
Recommended Citation
Jones S, Fox C, Gillam S, Gillam RB (2019) An exploration of automated narrative analysis via machine learning. PLoS ONE 14(10): e0224634. https://doi.org/10.1371/journal. pone.0224634
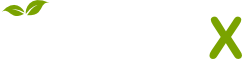
- Citations
- Citation Indexes: 10
- Usage
- Downloads: 283
- Abstract Views: 39
- Captures
- Readers: 46
- Mentions
- Blog Mentions: 1